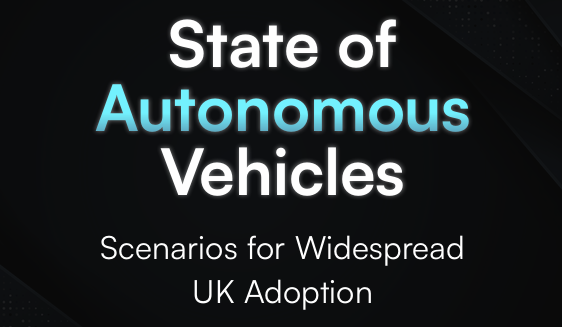
State of Autonomous Vehicles - June 2025 - Scenarios for Widespread UK Adoption
It has long been difficult to separate promise from reality, but with millions having now taken driverless rides in the US and China, AVs are no longer something of the future. The UK will start trials in London in Spring 2026. What does this mean for jobs and the way our cities are organised?
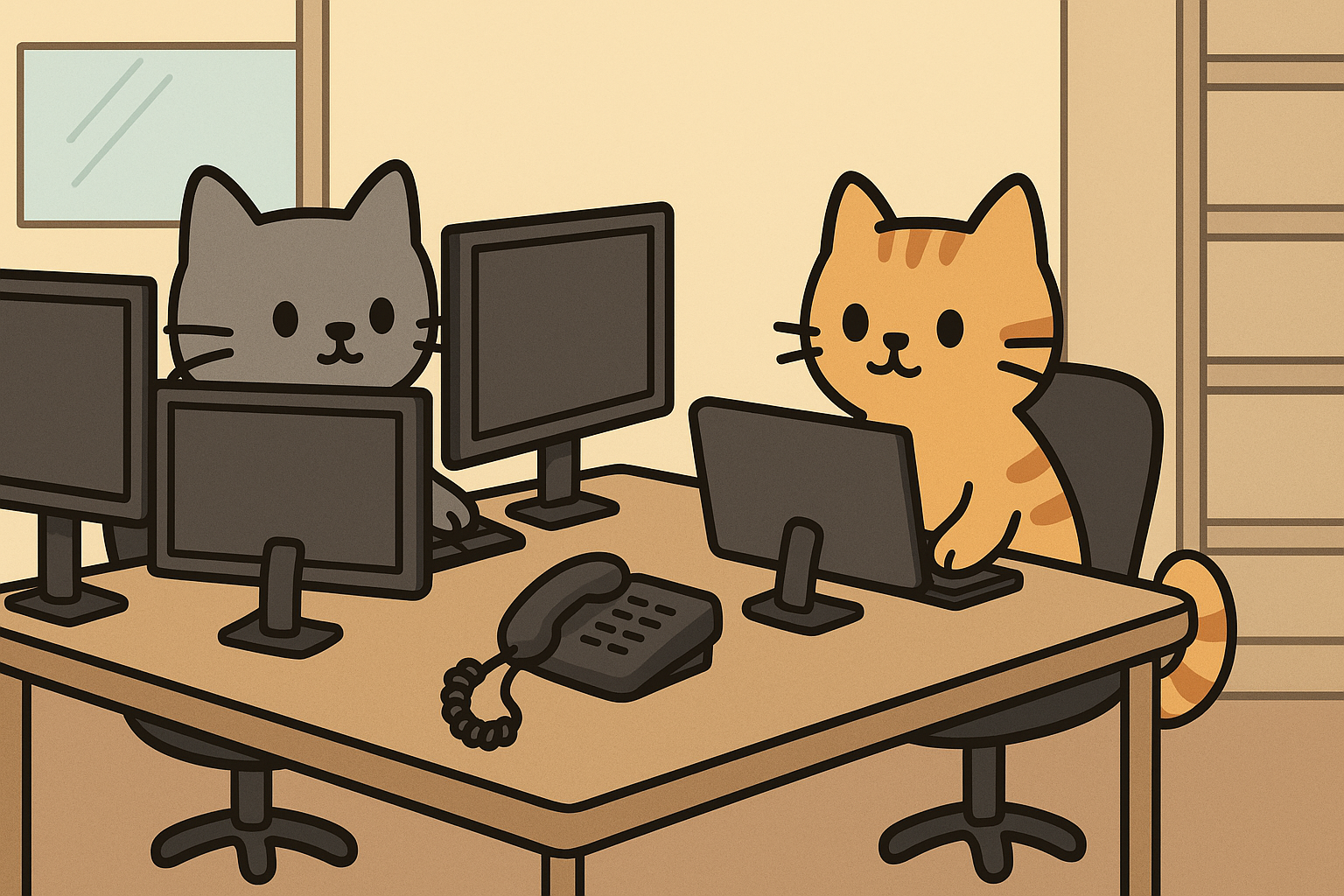
A Tale of Two ITies
A living collection of light, whimsical stories that show the challenges of integrating Corporate IT with the rest of the organisation

Infrastructure Flexibility in a Changing World: Lessons from Banking's COBOL Crisis
In an era of high business uncertainty - both technical and macroeconomic - preserving flexibility is more valuable than ever before.

State of Humanoid Robotics
Just as AI agents are rapidly automating knowledge work, physical automation through robotics represents the next frontier of labour transformation.